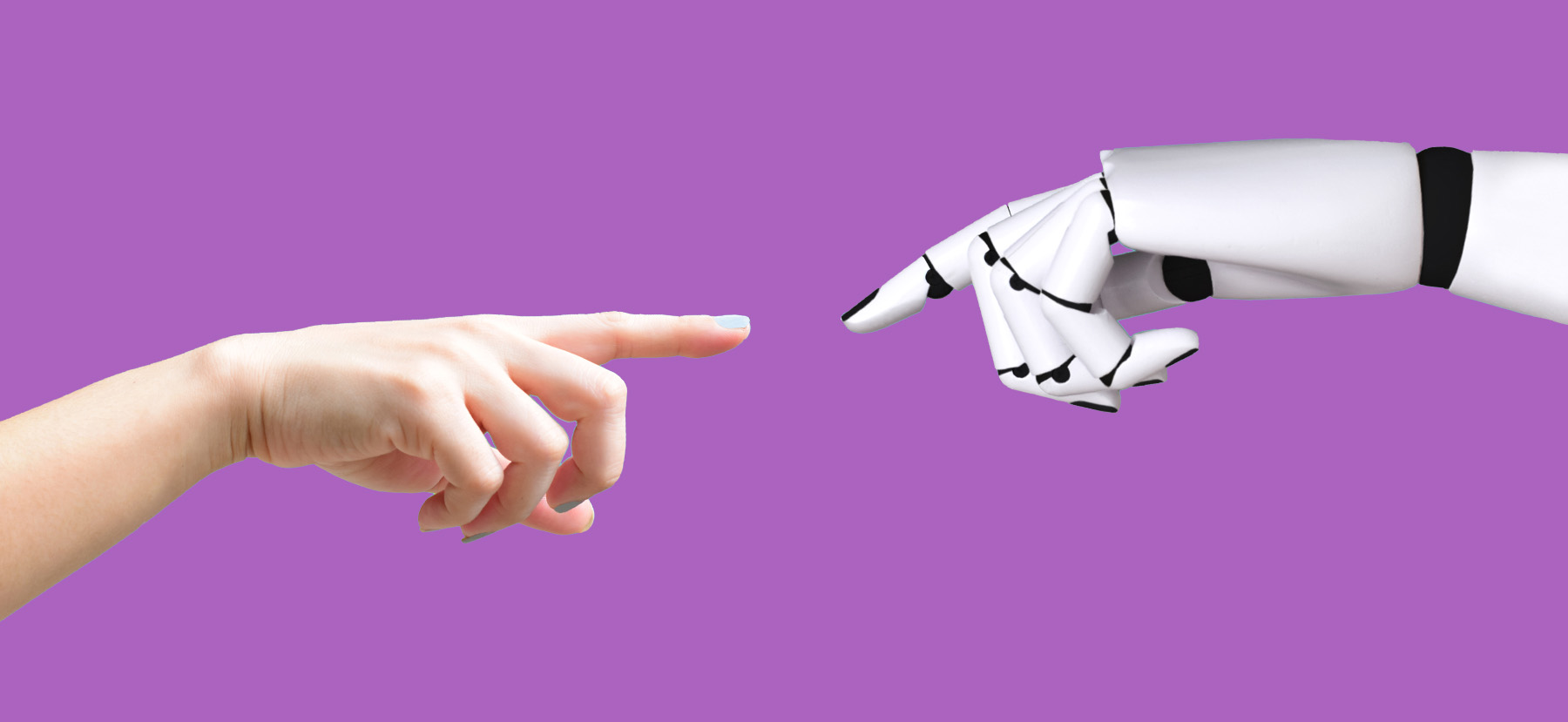
By Cheng Zhang, Ph.D., Jinwoo Kim, Ph.D., JungHo Jeon, Ph.D., Jinding Xing, Changbum R. Ahn, Ph.D., A.M.ASCE, Pingbo Tang, Ph.D., P.E., M.ASCE, and Hubo Cai, Ph.D., P.E., M.ASCE
Artificial intelligence, also called machine intelligence, is a powerful tool to address problems in the civil engineering field that traditional computational approaches cannot resolve. Although civil engineering projects can benefit from the accuracy and efficiency of AI in areas such as structural health monitoring, infrastructure sustainability analysis, optimization in structural design, construction safety monitoring, and disaster response, among others, there are also practical challenges that impede the further application of AI.
This practical use issue is especially the case when AI is employed in challenges related to the unique nature of civil engineering projects: their size, dynamic environment, large number of stakeholders, and other factors. These challenges can lead to noisy data, which are data that are either incomplete, contain errors, or are somehow less relevant to the analysis being conducted. In addition, some human-related data might not be available due to privacy issues.
Moreover, much civil engineering knowledge exists primarily in people’s minds, in the memories and experiences of the field’s professionals, rather than in other, more easily accessible resources. It can be difficult to integrate such personal knowledge into AI applications.
Current AI applications also can be hindered by poor explainability, which is the difficulty people might have in understanding how these models make their decisions or predictions. Consequently, many people lack trust in the results generated by AI. Finally, many people working in the civil engineering field have little or no coding experience, which causes difficulties in implementing and spreading AI-based solutions.
Considering other industries
To resolve these challenges, the civil engineering field needs to integrate human and machine intelligence by combining humans’ cognitive abilities, data mining, and the knowledge discovery capabilities of computers. How exactly this integration can be achieved is not yet known, partly because the civil engineering community lacks an in-depth understanding and systematic classification of its technical challenges.
These include the practical issues (such as social value, ethical considerations, privacy and policy issues, and business model considerations) and the existing knowledge gaps the community must overcome.
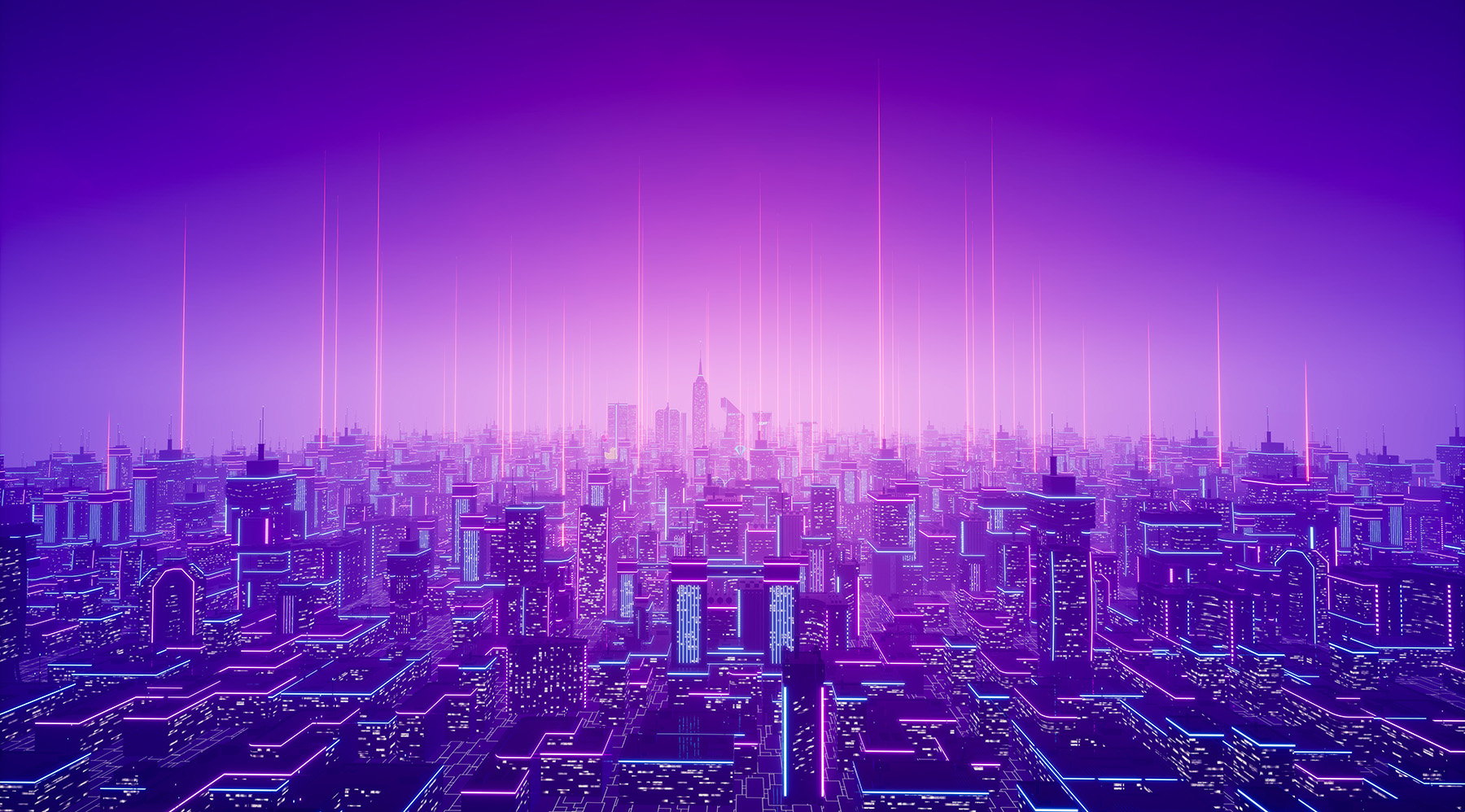
Although studies about the integration of human and machine intelligence in civil engineering are in the early stages, useful information can be gained from research conducted in other branches of science and engineering. In the field of medicine, for instance, a 2018 study (“Public Acceptability of Computer-Controlled Antibiotic Management: An Exploration of Automated Dosing and Opportunities for Implementation”) on the public’s confidence in AI-driven decisions found that people recognize the potential advantages of AI decision-making to improve the accuracy of a diagnosis. But members of the public also feel that interaction and oversight from a medical professional are necessary.
Likewise, in the field of transportation, research (“From Semi to Fully Autonomous Vehicles: New Emerging Risks and Ethico-Legal Challenges for Human-Machine Interactions”) indicates that AI can assist drivers in developing better risk awareness and implementing safer driving habits. But relying solely on AI in autonomous cars can result in fatal accidents because current AI applications are far from meeting the complex operational requirements of a dynamic traffic environment.
In construction safety management, the recent advent of sensing technologies (such as wearable sensors and vision sensors) generated various AI applications that identify or measure workers’ unsafe behaviors. These include the improper use of personal protective equipment, access to hazardous areas, and operating equipment in an unsafe manner.
But identifying at-risk workers and establishing interventions are delicate and complicated tasks that require the involvement of human intelligence because key safety-related indicators, including personal characteristics and safety attitudes, cannot be assessed solely based on sensory data.
There are various ways that the integration of human and machine intelligence can assist safety management. One is to incorporate machine intelligence measurement data into safety decisions while relying on human managers as the final decision-makers. Alternatively, human managers can feed input data sets, such as observation-based evaluations, into AI applications. Then the AI will identify at-risk workers by leveraging sensor-based data with the managers’ field observations. But this approach is very time- and labor-intensive for the human engineers.
Advanced anomaly detection systems at most nuclear power plants can identify faults in a single component/sensor or simultaneous faults in multiple components/sensors, relying on large amounts of sensor data. Experienced operators then investigate the anomalies’ root causes and generate recovery plans and prevention strategies.
Unfortunately, such efforts increase the human operators’ mental workloads, making it difficult to avoid human errors because of the rapidly changing data sources. Furthermore, other nuclear plant workers cannot record and reuse the knowledge and experiences generated in the human diagnosis process.
One promising approach is to analyze the human diagnosis process and use process mining algorithms to train anomaly detection systems that identify the root causes underlying the anomalies and recommend multiple recovery plans and prevention strategies. Released from laborious observation and control activities, the human operators are only responsible for choosing the proper control actions recommended by the AI, using their intuition, common sense, and contextual knowledge. This approach is complicated, though, because it is difficult to record the human operators’ decision-making process and train the AI using such data.
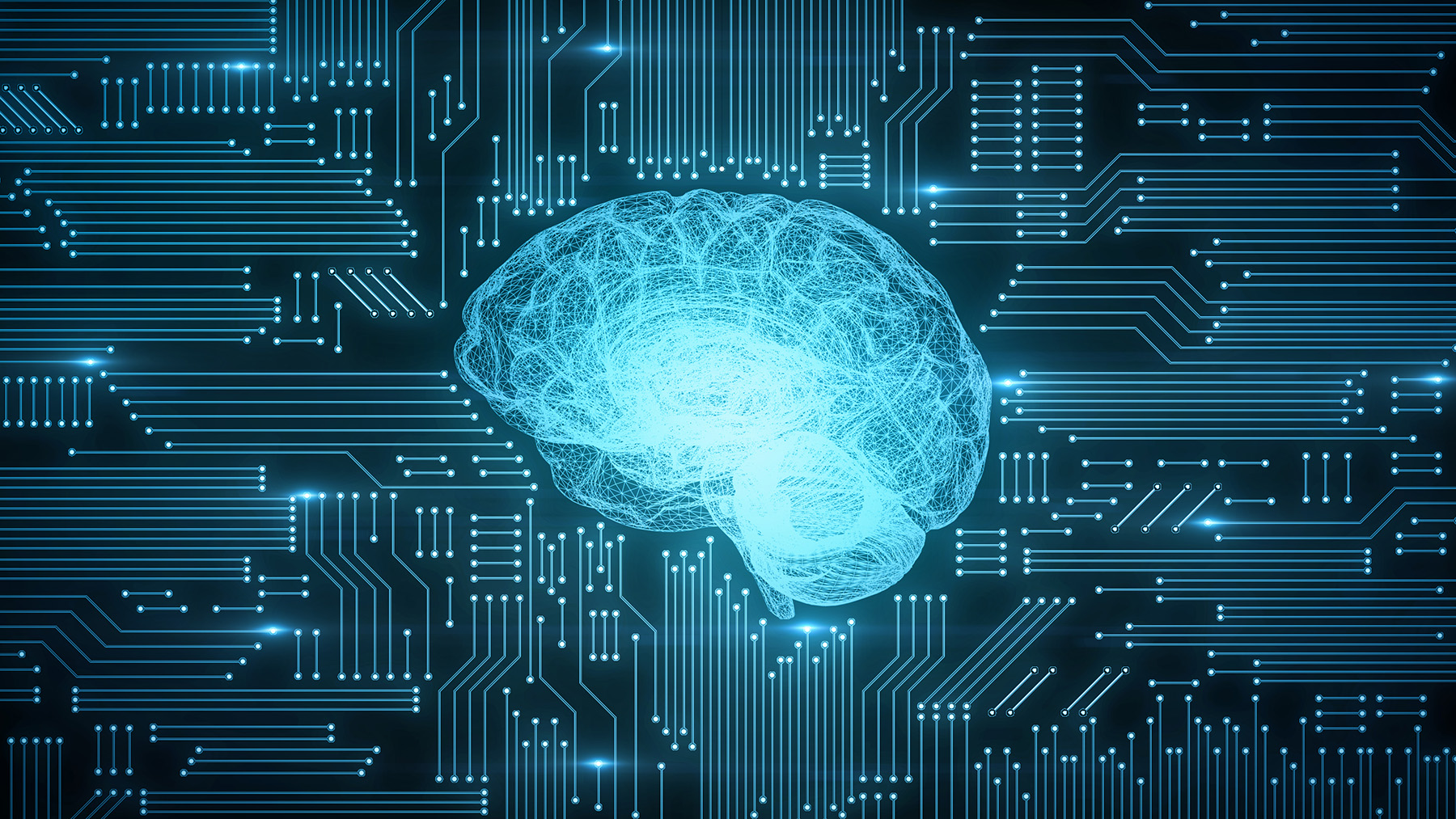
An analysis of existing literature on the integration of human and machine intelligence in civil engineering and a panel discussion — conducted by the authors in 2021 and involving researchers each with multiple years of experience in developing AI applications for civil engineering — make it clear that four critical gaps in knowledge exist regarding such integration. These knowledge gaps, in turn, helped generate a series of research questions that must be answered to advance the topic of integrated human and machine intelligence in civil engineering.
What are the civil engineering applications?
Civil engineering projects are complex and often involve the interactions of numerous entities, including the infrastructure itself, the environment, and multiple stakeholders who have different roles. Decision-making is a critical step that directly determines the success of a task.
While preliminary studies have illustrated the usefulness of AI in civil engineering, more attention should be paid to the configuration, balance, and trust between AI and humans in sharing the decision-making responsibilities in tasks of varying complexity. Consequently, it is unclear to researchers which tasks in the civil engineering field can be assisted/performed by AI and what role is appropriate for AI to assume — a massive hurdle toward maximizing AI’s value in civil engineering.
It is therefore paramount to characterize civil engineering tasks in terms of AI adaptability and configure the collaboration in decision-making between AI and humans. To help close this knowledge gap, the following questions need to be answered:
- What are the characteristics of the tasks that have benefited from AI?
- What are the tasks that may not benefit from AI?
- How can we use AI to make successful decisions on complex tasks?
How can people and machines interact?
AI can hone the efficiency and effectiveness of tasks for the architecture, engineering, and construction industry. At its most basic level, AI represents a system that can generate simple responses based on the given data, with a significant amount of those data labeled by humans. Labeling is the process by which people digitally annotate data — for example, by digitally marking images to identify which ones depict people or show safety hazards.
Unfortunately, the interface that allows a machine to learn from human inputs automatically and then adapt to the behavior of people is still in its infancy. As a result, human experts or AI system users often need to rely on data-driven approaches to adjust AI to support various human users effectively.
Such data-driven approaches can require people to manually label the data or record users’ latent metadata and decision-making processes while using AI. These human users might be experts who label and interpret the data for training the machine to complete similar data interpretation or AI system users who could interact with the computer for searching and finding the results.
Both types of interactions involve a significant need to capture and harness humans’ cognitive thinking abilities when they use AI applications. But it is unclear which human-AI interaction interfaces or communication protocols could support the harnessing of experienced operators’ decision-making processes and the training of AI applications in anomaly detection systems. To bridge this knowledge gap, the following questions must be answered:
- Who is using and/or will use AI for civil engineering projects?
- What interface should be used for AI in civil engineering to communicate with workers, managers, engineers, and other professionals?
- What verbal and nonverbal communication protocols are needed between humans and machines?
- Which safety issues will be raised by human and AI integration in the civil engineering field? Especially, how might such integration affect the psychological health of human workers?
- How can we ensure transparency and explainability behind the decision-preparation processes used by AI so that they match the cognitive capacities of human decision-makers?
What are the barriers to data collection?
The collection of data to help integrate human and machine intelligence applications in civil engineering poses particular challenges because of the type and complexity of problems that must be tackled. Complex problems and high-level decision tasks usually involve what is called secondary data — including historical project data such as daily work reports, production rates, and work change orders — generated from project stakeholders (including designers, subcontractors, construction managers, and workers) rather than raw sensory data.
Thus, the availability of data is limited. Furthermore, collecting such data can pose confidentiality and privacy issues.
In addition, data annotation applications often require significant field-specific knowledge and the collective decisions of multiple experts. For example, the applications for detecting construction safety violations would need to be annotated for unsafe scenarios, with such determinations requiring the consolidation of the judgments of multiple experts.
So, it is critical to know which types of human-machine intelligence integration methods are effective with the data available and what trade-offs might be necessary between the data availability and the performance of the integration model. However, such knowledge is still limited in civil engineering; thus, more research is required to optimize data collection efforts.
To bridge these knowledge gaps and identify the most reliable, safe, and efficient approaches to collecting the necessary data through human-AI interfaces, the following questions should be answered:
- What data are generated in civil engineering tasks?
- What types of data does AI used in civil engineering need to process?
- What are the most efficient ways to process structured data (spreadsheets, databases, graphs, etc.) versus unstructured data (text, imagery, sound, sensor data, etc.)?
- What is the best way to annotate data requiring civil engineering knowledge and then use those data to train AI?
What are the impacts on industry and society?
Finally, what are the broader impacts posed by the integration of human and machine intelligence for the AEC industry and society as a whole? The existing literature features extensive discussions about the impact of AI and automation on the economy at the macro level, with researchers generally agreeing that AI will stimulate economic development while its effects on specific industries will vary. For example, AI will likely have a complex effect on the job market, perhaps increasing overall productivity while also inducing labor disruptions.
Focusing on a micro point of view, it is unclear what the impact will be of integrating human and machine intelligence on individuals, especially employers and employees in specific fields such as civil engineering. What jobs may disappear, what new jobs may emerge, what new tasks will appear in existing jobs, and what new skills will people need to develop?
If we can correctly identify or predict these impacts, we may be able to develop policies to help the civil engineering field better embrace the opportunities and mitigate the threats of the integration of human and machine intelligence. But finding the answers is difficult because it requires data that generally are available only after the impact has occurred, causing irretrievable harm to the economy, industry, and society.
To bridge this knowledge gap and support a healthy labor market for the AEC fields requires answers to the following questions:
- How will human-AI integration change the job market? How can we manage the reduced availability of low-skilled jobs that will result from increasingly capable machines?
- How will human-AI integration change the interests of stakeholders, the AEC industry, and education in the science, technology, engineering, and mathematics fields?
The road ahead
Looking forward, the efficiency and high consistency of machine intelligence combined with the adaptability of human decision-makers can provide many benefits on civil engineering projects. But first, a series of critical questions need to be addressed, ranging from which civil engineering tasks can benefit the most from AI to what the ultimate impact of AI will be on the larger society in which civil engineers operate.
Key barriers to data collection must also be overcome as new approaches must be developed to enable machine intelligence to use such data.
So perhaps the only certainty in all this is how much work remains to be done. During 2022, for instance, we worked with distinguished scientists from the fields of computer science, human factors, and human-computer interaction to share their insights into the topic of integrated human and machine intelligence.
We also collaborated with researchers, practitioners, and software developers to better understand their perception of how integrated human and machine intelligence can affect the civil engineering field.
These and other efforts have unearthed intriguing topics that demonstrate the application of integrated human and machine intelligence in numerous civil engineering-related areas, including:
- Human-robot collaboration in construction.
- Project management, planning, and risk analysis.
- Trust and ethical considerations in AI applications.
- Construction material and quality analysis.
- Design and educational methodologies.
- Safety protocols and ergonomic design.
Such research activities highlight the promising future and widespread applicability of integrated human and machine intelligence in propelling advancements in civil engineering.
Cheng Zhang, Ph.D., is an assistant professor in the Department of Construction Science & Organizational Leadership at Purdue University Northwest in Hammond, Indiana. Jinwoo Kim, Ph.D., is an assistant professor in the Department of Architectural Engineering at Gachon University in Seongnam, South Korea. JungHo Jeon, Ph.D., is an assistant professor in the Department of Civil and Environmental Engineering and Engineering Mechanics at the University of Dayton in Ohio. Jinding Xing is a Ph.D. candidate in the Department of Civil and Environmental Engineering at Carnegie Mellon University in Pittsburgh. Changbum R. Ahn, Ph.D., A.M.ASCE, is an associate professor in the Department of Architecture and Architectural Engineering at the Seoul National University in South Korea. Pingbo Tang, Ph.D., P.E., M.ASCE, is an associate professor in the Department of Civil and Environmental Engineering at Carnegie Mellon University. Hubo Cai, Ph.D., P.E., M.ASCE, is a professor in the School of Civil Engineering at Purdue University in West Lafayette, Indiana.
Selected references
Bellet, T., Cunneen, M., Mullins, M., Murphy, F., Pütz, F., Spickermann, F., Braendle, C., & Baumann, M.F. (2019). From semi to fully autonomous vehicles: New emerging risks and ethico-legal challenges for human-machine interactions. Transportation Research Part F: Traffic Psychology and Behaviour, 63(May): 153-164. https://doi.org/10.1016/j.trf.2019.04.004Rawson, T.M., Ming, D., Gowers, S.A., Freeman, D., Herrero, P., Georgiou, P., Cass, A.E., O’Hare, D., & Holmes, A.H. (2019). Public acceptability of computer-controlled antibiotic management: An exploration of automated dosing and opportunities for implementation. Journal of Infection, 78(1): 75–86. https://doi.org/10.1016/j.jinf.2018.08.005
This article is based on the paper, “Toward Integrated Human-Machine Intelligence for Civil Engineering: An Interdisciplinary Perspective,” presented at the ASCE International Conference on Computing in Civil Engineering 2021, held in Orlando, Florida, Sept. 12-14, 2021.
This article first appeared in the September/October 2023 print issue of Civil Engineering as “Human-Machine Intelligence: Opportunities and Obstacles.”