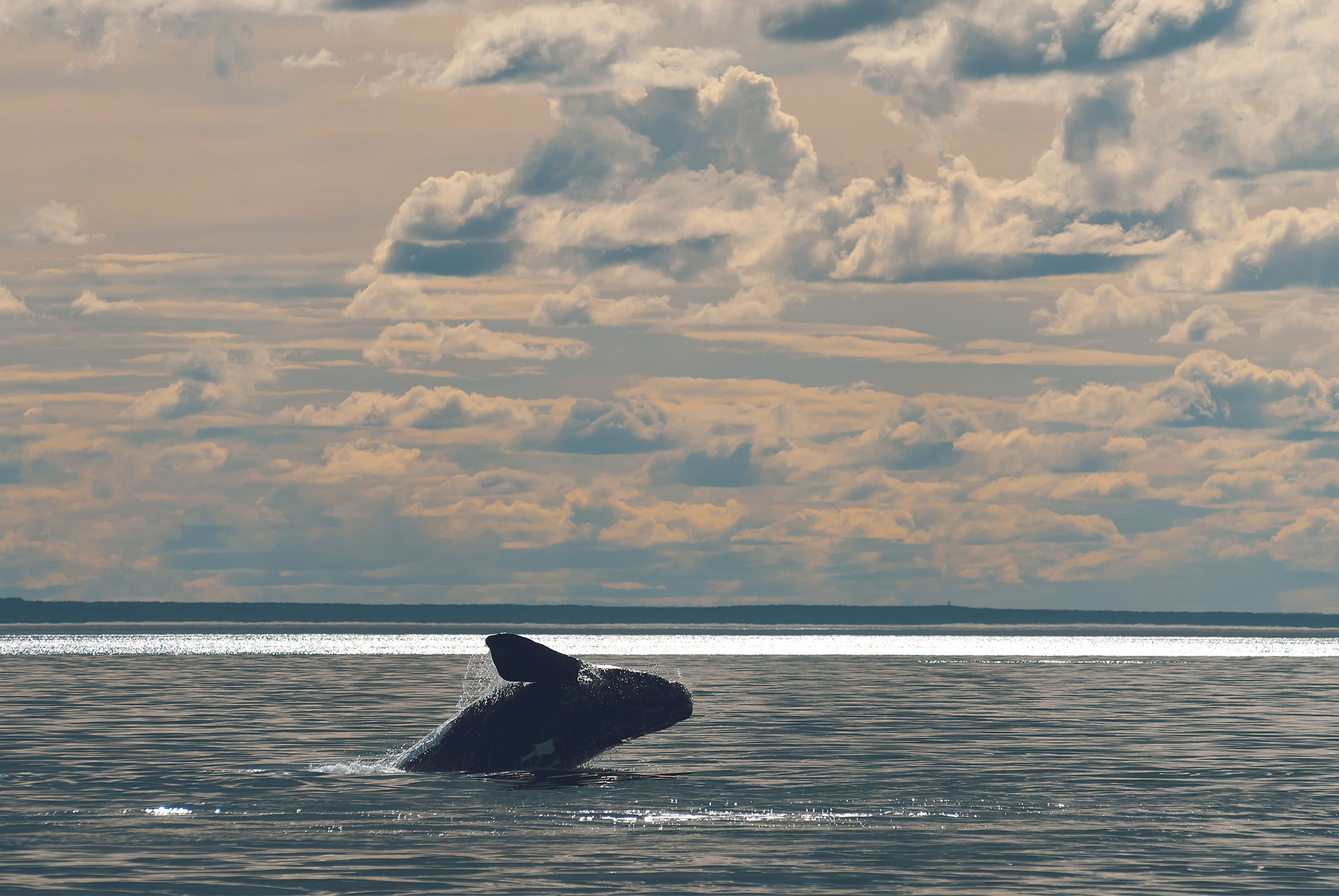
By Kayt Sukel
With increased investment in offshore wind turbines, the state of New Jersey was poised to begin several high-profile renewable projects along its southern shoreline. Yet many groups opposed the new energy developments, citing concerns about the North Atlantic right whale, which migrates to waters near the Jersey shore each winter. With the uptick in interest in offshore wind, Ahmed Aziz Ezzat, Ph.D., an assistant professor in Rutgers University’s Department of Industrial and Systems Engineering, said he and his colleagues were inspired to create a high-resolution model to help predict where and when this critically endangered species might be found.
“We were really interested in seeing if we could help inform the development and construction of these offshore wind farms in a way that is as environmentally friendly as possible,” he explained. “Could we collect the right data to help developers, as well as other stakeholders in the blue economy, better understand the risks of where and when they might encounter a particular type of marine mammal?”
To do just that, Ezzat partnered with Josh Kohut, Ph.D., professor in the department of marine and coastal sciences and dean of research at Rutgers’ School of Environmental and Biological Sciences. Together they created a new artificial intelligence/machine learning algorithm, which they refer to as AI/ML, to help predict the right whale’s migration path and habitat, with the goal of informing more responsible design of offshore wind farms, as well as more conscientious, conservation-based ocean development.
“Having tools like this could serve to support mitigation strategies ... so there’s some real practical need,” said Kohut. “It’s very useful for planning purposes, when you talk about responding or reacting within a regulatory framework.”
Finding a needle in a haystack
It is often said that North Atlantic right whales get their name for being a popular target for whalers in the 19th century. Whether or not this naming explanation is correct, these whales were, as Kohut put it, the “right” whale to go after. “They spend a lot of time on the surface, they’re slow swimmers, in general, and when they are killed, they float, which makes it much easier to process the whale,” he said.
As a result, the species was decimated during whaling’s heyday. While the practice of whaling has now been outlawed in most places, it has been difficult for this particular species to recover, even from losses sustained more than a hundred years ago. They do not breed every season — and now they face other deadly hazards in their habitats, including vessel strikes and fishing gear entanglements, which, by last count, reduced their numbers to somewhere between 350 and 370 living animals, according to Kohut.
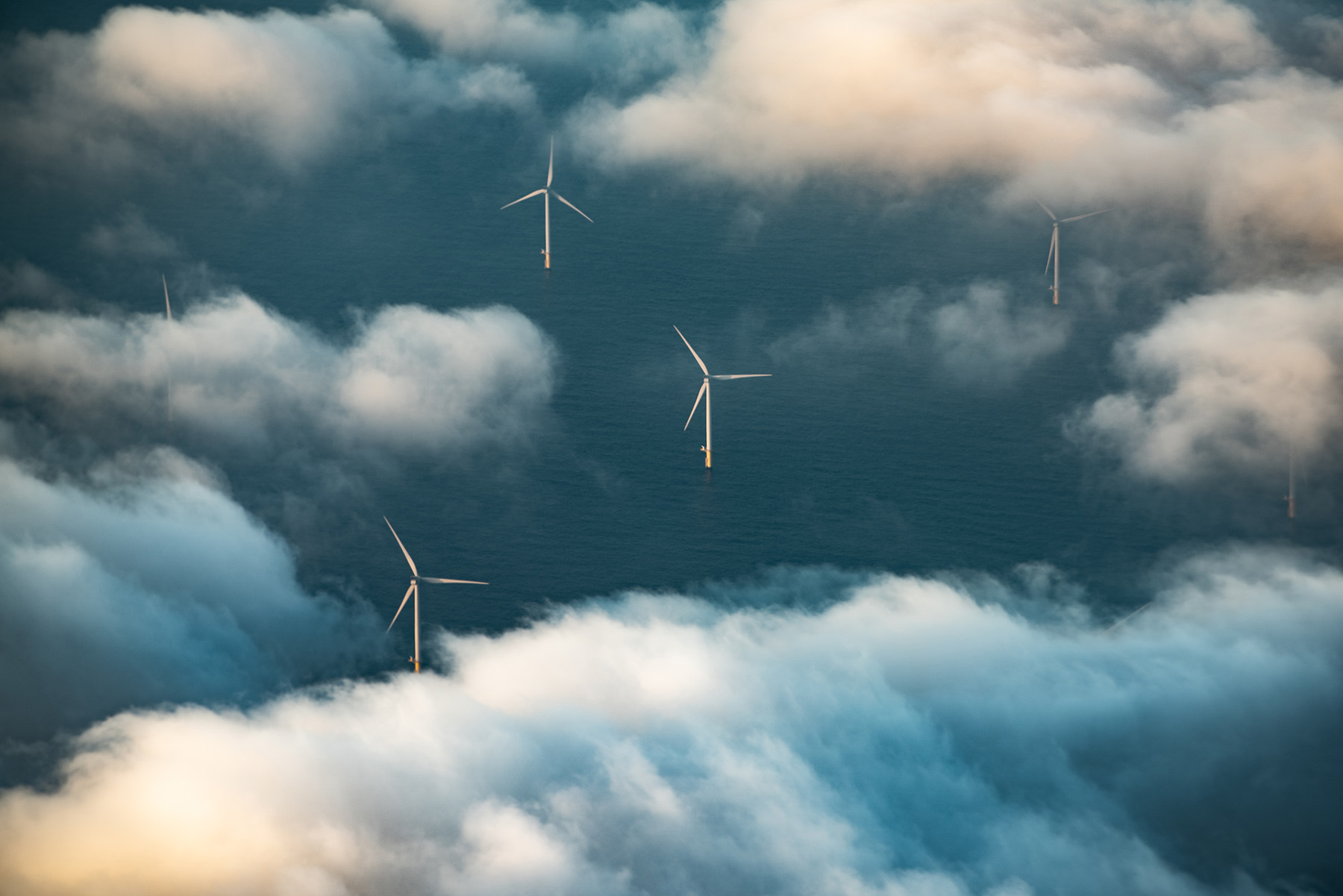
As environmental groups and researchers have looked to protect the right whale, they’ve asked for better ways to track them in order to more efficiently predict their location and migration paths. Rob Schick, Ph.D., a research scientist at Duke University, said aerial and vessel surveys have been hugely important in this work.
“This forms the crux of a lot of the big, range-wide habitat modeling that goes on,” Schick explained. “Then we also have satellite tracking data.”
Images from surveys and satellites give researchers a vague idea of where right whales may be. In addition, some research groups also use passive acoustic techniques to track these underwater mammals. These techniques leverage sensors placed on fixed platforms on the seafloor to identify the whale’s tell-tale singing. Those sensors may also be deployed on underwater gliders (autonomous, unmanned underwater vehicles) to pick up whale calls, as well as other high-resolution data about the areas that whales may be visiting, according to Ezzat.
“What was the state of the ocean at the time the whale was detected? What was the ocean color? What was the sea surface temperature? What were the other environmental variables?” said Ezzat. “By looking at these very high-resolution data over space and time, AI can possibly unlock what classical ecological models cannot do.”
Leveraging multimodal data
Historically, Schick said, researchers have had difficulty populating those classical ecological models with myriad data types. Different studies use “different kinds of observational modalities,” each with their own pros and cons, depending on the specific research question they are trying to answer. That has made it hard to come up with a robust model to accurately predict where right whales may be at any given moment.
“There’s just this huge pool of data and they all kind of tell a different story about where the species is at any different time, or where they may have been,” Schick said. “It’s a huge challenge, certainly in our right whale world, but also in habitat modeling in general, to try to fuse or bring together multiple data sets because they all sort of give you an imperfect picture of what’s really going on.”
Enter AI/ML. Ezzat said that ML algorithms can look for patterns across all these different data points, bringing them together in sometimes unexpected ways to correlate the position of a right whale under different environmental conditions. As more data points come in, the algorithm adjusts to improve its predictions. Ezzat said their model, which leverages data from both underwater glider and satellite images, provides a probability map as a result, offering users information about the statistical likelihood of encountering a right whale at a specific place and time.
“Through this high-resolution model, if we’re able to get a highly localized prediction of where and when you would encounter an endangered marine mammal like the North Atlantic right whale, you can think of many ocean users that could benefit from such predictions for different reasons,” Ezzat said.
The information could prove important not just to offshore wind development, Ezzat added, but to other blue economy players, especially as they work to comply with stringent environmental regulations.
Kohut said that’s the exciting part.
“This collaboration has afforded us the ability to think about ways in which we can make it more of a real-time tool for decision making and compliance with regulatory set requirements,” he explained. With the model’s results in hand, vessel operators, for example, will know there is a high likelihood of encountering a right whale at certain time points or during particular environmental conditions. They can then exercise caution to avoid them.
Expanding the model
With the success of their initial model, Ezzat and Kohut are excited to include new data to further refine its right whale location predictions. They would also like to leverage AI/ML to locate other mammals and ocean species in the future.
“We’re constantly looking for other external data sets that we can feed into our model and possibly improve our predictions,” said Ezzat. “One that we talk about all the time is to incorporate prey information. A lot of preferences for North Atlantic right whale can be potentially explained in light of foraging.”
Kohut added that with new data becoming available, they can also refine the kind of environmental data they use. “Instead of looking at a map of sea surface temperature, where maybe species prefer certain temperatures of water, we can look at where the fronts are in those maps, where the gradients are, and find out how important those gradients are,” he said.
Schick said he is heartened by these kinds of advances, and he hopes that offshore wind developers and other ocean economy players will be able to rely on more than just “broad-scale density patterns” as they make decisions in the future to better protect endangered species and work with a greater conservation focus.
“Doing data simulation with multiple data streams in a deep learning framework, or even just a standard sort of statistical framework, is really key,” Schick said. “I’m optimistic.”
Kayt Sukel is a science and technology writer based outside Houston.
This article first appeared in the May/June 2025 issue of Civil Engineering as “A Whale of an Algorithm.”