Identifying Pathological Variations in In Vivo Right Ventricular Mechanics
University of Pittsburgh
Problems
A core research area of the Computational Diagnostics and Inverse Mechanics (CDIM) Group at the University of Pittsburgh is the development of new technologies and evaluation of new discoveries in the area of heart mechanics in relation to changes caused by pulmonary hypertension (PH). PH is a cardiopulmonary disease, where if left untreated, is devastating with poor long-term prognosis. With early detection several treatments have been found to be successful in vastly improving prognosis, but diagnosis is often delayed because the early symptoms of PH are nonspecific. One particular unifying observation about PH is that adaptation of the right ventricle (RV) under the sustained pressure overload is a major determinant of patient symptoms and, more importantly, survival. Furthermore, the RV with PH dilates significantly over time, becomes more concentric, and the septum flattens. Without treatment the RV will further dilate over time and eventually fail. Yet, while a connection between RV mechanical function (e.g., shape, kinematics, material properties, etc.) and PH clearly exists, there is still no clear understanding of why certain patients with severe PH maintain near normal cardiac outputs for long periods of time, and others experience a more rapid deterioration leading to RV failure.
Approach
Our approach has been focused on developing methods to utilize the current standard-of-care cardiac medical imaging to evaluate changes in the mechanical function of the RV related to PH, and which have the potential to enable new levels of diagnosis and prognosis. Initially, we have examined the shape and kinematics of the RV, with the overall process being developed described as follows: (a) RV surface extraction from medical imaging for each patient at each phase in the cardiac cycle. (b) Topological mapping of all RV surfaces to a common domain and registration (i.e., build correspondence between RV shapes). (c) Statistical decomposition of RV shapes into fundamental features (i.e., modes). (d) Clustering and classification of RV shape features with respect to the hemodynamic state. More recently, we have been extending this work to utilize the shape analysis within an inverse characterization routine to estimate the RV wall stiffness noninvasively and nondestructively (i.e., again only utilizing the imaging data).
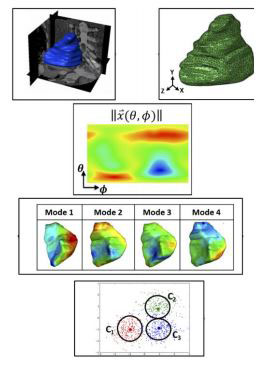
Findings
We developed a novel computational approach involving direct topological mapping combined with statistical decomposition that better maintained anatomical consistency for three-dimensional (3D) shape analysis compared to other existing approaches and was applicable to any patient set and imaging modality. We also examined three-dimensional shape analysis applied to the right ventricle as a proof-of-concept for the purpose of understanding and classifying changes due to pulmonary hypertension. The analysis of a set of human hearts showed clear evidence that the shape/kinematic features that could be obtained with our statistical shape analysis procedure were anatomically relevant and intimately linked to the state of pathology in the heart. Lastly, in one of our more recent efforts, we firstly formulated and presented a generally applicable approach to use independent component analysis (ICA) instead of the traditionally used principal component analysis (i.e., proper orthogonal decomposition) for 3D statistical shape analysis. In addition to presenting the novel algorithm, we showed that this new approach for 3D statistical shape analysis is unique compared to the traditional approach, and can provide an alternate view of the function of a biological structure and its relevance to pathological conditions.
Core competencies
- Algorithms for Inverse Problems in Applied Mechanics and Engineering (including material characterization, optimal design, etc.)
- Computational Mechanics
- Applied Numerical Optimization
- Applied Machine Learning and Pattern Recognition
- Reduced-Order Modeling
Current research team members
- Mohammad Ahmadpoor (Ph.D. Candidate)
- Gregory Banyay (Ph.D. Student)
- Jing Xu (Ph.D. Student)
- Matthew Grasinger (Ph.D. Student)
- Robert Zupan (Ph.D. Student)
- Erin Sarosi (undergraduate)
- Rahul Rege (undergraduate)
- Abraham Umo (undergraduate)
Current core research projects (and core collaborators)
- Evaluation of RV Mechanical Function (Marc Simon and Timothy Wong, UPMC, and Wilkins Aquino, Duke)
- Noninvasive/Nondestructive Dynamic Methods for Tissue Characterization (Matthew Urban, Mayo Clinic, and Kang Kim, UPMC)
- Smart Material, Adaptive, and Reconfigurable Building Tiles (Richard Beblo, UDRI, and Dale Clifford, CalPoly)
- Assessment of Wellbore Cement Integrity (Julie Vandenbossche and Anthony Iannacchione, Pitt)
- Reduced Order Modeling for Simulating Rector Thermal-Fluid Processes (Mark Kimber, Pitt)