Uncertainty Modeling & Propagation in Complex Dynamic Systems
Columbia University
Problems
"… The ubiquity of uncertainty in computational estimates of reality and the necessity for its quantification… " has been recently recognized by the National Academies and the National Research Council. In this regard, a large portion of the engineering mechanics/dynamics community has focused on multi-scale/multi-physics problems with stochastic media properties, random excitations and uncertain initial/boundary conditions. Two main challenges associated with uncertainty treatment relate to the (A) modeling, and the (B) propagation of the uncertainties.
Addressing challenge (A) relates to the development of methodologies for the interpretation/analysis of measured available data, as well as for the estimation of pertinent stochastic models, i.e. quantification of the underlying stochastic process/field statistics. First, measured data most often exhibit a time/space-varying behavior; thus, statistical estimators based on joint time/space-frequency analysis tools (e.g. wavelets) need to be developed. Second, multi-scale computational analyses necessitate the development and application of multi-scale statistical descriptors capable of capturing complex uncertainty relationships across scales. Third, most often there are limited, incomplete and/or missing data; thus, techniques are required not only for estimating stochastic process/field statistics subject to vastly sparse/limited/incomplete data, but also for quantifying the uncertainty of the estimates as well.
Addressing challenge (B) relates to the development of methodologies for determining response/reliability statistics of complex systems, i.e. development of analytical and/or numerical methodologies for solving high-dimensional nonlinear stochastic (partial / fractional) differential equations efficiently. First, Monte Carlo Simulation (MCS) has been the most versatile approach for addressing this challenge. However, it can be computationally prohibitive for relatively large-scale complex systems, or when the quantity of interest has a small probability of occurrence (e.g. failure probability); thus, there is a need for developing efficient analytical/numerical solution methodologies. Second, the ever-increasing available computational capabilities, as well as advanced experimental setups have contributed to a highly sophisticated modeling of engineering systems and related excitations. As a result, the form of the governing dynamics equations has become highly complex from a mathematics perspective (e.g. generalized/fractional calculus). Clearly, the solution of such equations has become a much more challenging task than it used to be a decade ago.
Approach
Recent and ongoing work to address challenge (A) relates to the development of a versatile framework for spectral analysis and stochastic process/field statistics quantification subject to vastly sparse/limited/incomplete data based on the emerging concept of compressive sensing, and on joint time-frequency analysis tools such as wavelets. Preliminary work indicates satisfactory accuracy for up to 80% missing data.
Recent and ongoing work to address challenge (B) relates to the development of a general and robust computational framework, based on functional/path integrals theory, for stochastic analysis of complex engineering mechanics/dynamics systems. Preliminary work indicates that functional integrals exhibit significant accuracy, and efficiency (in terms of computational cost) and can be, potentially, a promising alternative to computationally demanding MCS.
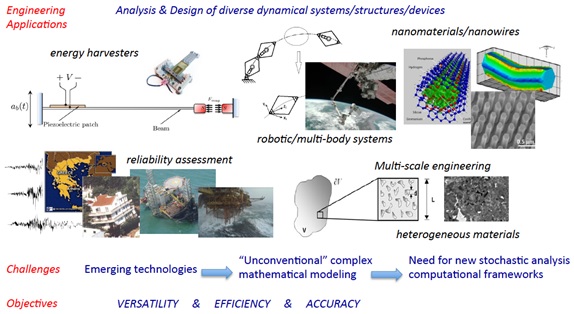
Impact
Findings from this research will have a major impact on the analysis and design of diverse dynamical systems/structures/devices, and will open major roadblocks and unlock a vast array of applications in emerging and transformative technologies such as in the areas of energy (e.g. analysis/design of energy harvesters, batteries), nano-materials (e.g. nanowire dynamics), robotic/multi-body system dynamics, and multi-scale engineering (e.g. homogenization and uncertainty propagation from the nano- to the macro-scale).
Core competencies
- Nonlinear Stochastic Dynamics
- Computational Stochastic Mechanics
- Uncertainty Quantification Methodologies
- Signal Processing Techniques
- Diverse Applications in Civil Engineering & Engineering Mechanics
Current & past research group members
- Olga Brudastova (PhD student)
- Liam Comerford (Post-Doc, U. Hannover)
- Ketson dos Santos (PhD student)
- Vasileios Fragkoulis (PhD student)
- Antonios Meimaris (PhD student)
- Ioannis Mitseas (Post-Doc, U. Hannover)
- Apostolos Psaros (PhD student)
- Yuanjin Zhang (PhD student)